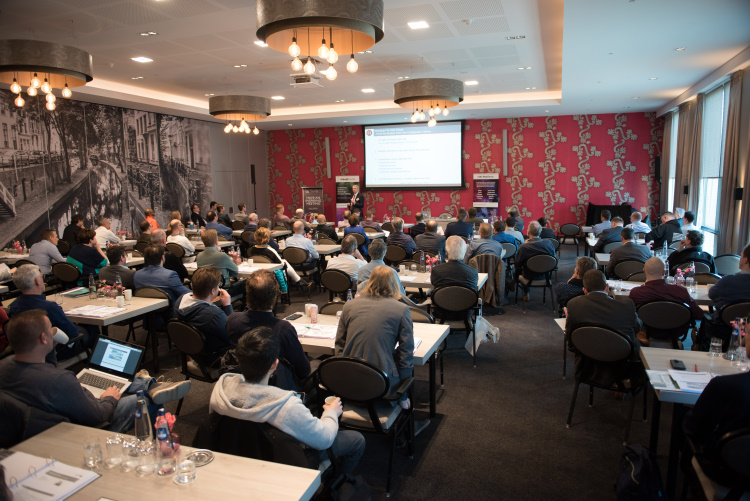
[DE WORKSHOPS OP 3 APRIL ZIJN ENKEL LIVE IN UTRECHT.]
Innovatie binnen wettelijke kaders: de AI Act, Data Governance Act en Data Act
In een tijd waarin data en kunstmatige intelligentie een centrale rol innemen in bedrijfsstrategieën, is het cruciaal om niet alleen de nieuwe mogelijkheden, maar ook de nieuwste Europese wetgeving op dit gebied te begrijpen. Deze sessie biedt een boeiende en toegankelijke blik op de drie meest invloedrijke Europese wetten van dit moment: de AI Act, de Data Governance Act en de Data Act. Wat betekenen deze wetten voor uw organisatie, en hoe kunt u innovatie stimuleren terwijl u voldoet aan complexe wettelijke eisen? Met een unieke combinatie van technische en juridische expertise worden de belangrijkste onderdelen van deze wetten toegelicht, met daarbij praktische inzichten om organisaties toekomstbestendig te maken. U kunt rekenen op een informatieve sessie vol concrete tips, valkuilen en praktijkvoorbeelden.
- Een overzicht van Europese wetgeving in het digitale domein
- De AI Act – wat is verboden en hoog-risico AI?
- De Data Governance Act – hoe delen we data op een betrouwbare, transparante en ethische wijze?
- De Data Act – hoe regelen we toegang tot data, met name voor IoT, om een eerlijke en competitieve data-economie te realiseren?
- Praktische tips om juridisch compliant te blijven zonder innovatie te beperken.
Datawarehouses bouwen met GenAI: Een Toekomstblik
Data engineers zijn schaars, maar stel je voor dat je met GenAI zelf een data warehouse kunt bouwen! Victor de Graaff, oprichter van D-Data, zal in deze inspirerende sessie laten zien hoe het mogelijk is om zonder diepgaande technische kennis in slechts 45 minuten een compleet datawarehouse op te zetten, te vullen en er een BI-dashboard bij te maken.
Victor demonstreert dit proces met behulp van openbare APIs en GenAI, waarbij hij de kracht van automatisering en kunstmatige intelligentie inzet. In deze showcase staan Azure en ChatGPT hem bij als ‘digitale assistenten’, en maken ze het onmogelijke mogelijk.
Door GenAI-gegenereerde code te gebruiken, zullen we:
- Een datawarehouse aanmaken en configureren zonder ingewikkelde scripts
- Data direct vanuit publieke APIs ophalen en laden
- Deze data visualiseren in een overzichtelijk BI-dashboard
Deze sessie laat zien dat zelfs specialistische taken, zoals het bouwen van datawarehouses, bereikbaar zijn voor een breder publiek dankzij GenAI. Bereid je voor om “in awe” te zijn en ontdek de toekomst van BI en data-engineering met kunstmatige intelligentie!
Lees minderTesten in een BI & Data landschap
Onze dataprocessen en -systemen worden steeds complexer en dynamischer. Veel bedrijven hebben moeite met het op peil houden van de datakwaliteit en het vergroten van het vertrouwen in het datalandschap.
Testen biedt inzicht in risico’s en kwaliteit van de data, de systemen en de datastromen. Het onderzoekt bijvoorbeeld de prestaties, de data-integriteit en de bedrijfslogica. Veel meer dan het vinden van problemen en bugs gaat het bij testen om het bieden en opbouwen van vertrouwen voor eindgebruikers in de oplossing die wordt gebouwd. Testen zou daarom een cruciaal onderdeel moeten zijn van elke business intelligence- en data omgeving.
In deze lezing ga ik in op testkennis gericht op data omgevingen met behulp van TMAP en het VOICE-model. Ik zal ingaan op de DAMA-kwaliteitskenmerken die je kunt overnemen en je aanmoedigen om het niveau van vertrouwen in de kwaliteit van uw systemen en gegevens te communiceren. Krijg inzicht en tips over het testen van BI- & Data-oplossingen.
Belangrijkste punten:
- Het belang van testen
- Het TMAP- en VOICE-testmodel
- Vertrouwen opbouwen door inzicht te geven in het kwaliteitsniveau
- Testen in een BI & Data omgeving door te kijken naar:
- Data stromen; kijken naar hoe de data door het systeem heen beweegt
- Data kwaliteit; welke KPI’s kunnen worden gebruikt?
- Data Profiling; hoe je bugs kunt vinden nog voordat de oplossing is gebouwd.
Onderzoek Vernieuwen door Open Data: Het Bouwen van het Samenwerkingsplatform van de toekomst
Erasmus Universiteit en TU-Delft hebben in 2023 de krachten gebundeld om een nieuw tijdperk van onderzoekssamenwerking in te luiden met een innovatief platform voor het delen van (open) data. Gebouwd op de pijlers van gebruiksgemak, robuuste beveiliging en moderne infrastructuur maakt dit platform het delen en ontdekken van onderzoeksgegevens een fluitje van een cent. Onderzoekers profiteren van intuïtief databeheer met geautomatiseerde Digital Object Identifier (DOI) toekenning, terwijl geavanceerde beveiliging zorgt voor AVG-compliance zonder de toegankelijkheid in gevaar te brengen. Het platform biedt geautomatiseerde datasynchronisatie en unieke compute-to-data mogelijkheden, waardoor algoritmes veilig kunnen worden uitgevoerd met behoud van gevoelige informatie.Als open-source oplossing stimuleert het platform actieve deelname van de gemeenschap en continue verbetering. Of u nu een bank bent die markttrends analyseert, een verzekeraar die risico-inzichten zoekt, of een retailer die klantgedrag onderzoekt: ontdek hoe dit platform veilige datacollaboratie mogelijk maakt terwijl uw intellectueel eigendom wordt beschermd en u volledige controle houdt over uw gevoelige informatie.
Deze sessie zal het volgende belichten:
- Platform Architectuur: Ontdek de bouwstenen van een modern datadeelplatform met focus op beveiliging en gebruiksgemak.
- Praktische Toepassing: Leer hoe organisaties data kunnen delen met volledige controle over hun gevoelige informatie.
- Technische Realisatie: Verken de implementatie van beveiligingsmaatregelen en geautomatiseerde functies voor efficiënt datadelen.
- Community Ontwikkeling: Begrijp hoe je een actieve datagemeenschap opbouwt tussen kennisinstellingen en bedrijfsleven.
- Toekomstbestendigheid: Zie hoe open-source ontwikkeling zorgt voor continue innovatie en AI-gereedheid van het platform.
Federated Computational Data Governance - toepassing in de praktijk
Hoe kun je data echt inzetten als bedrijfsmiddel? We verkennen de centrale pijler van Data Mesh: Federated Computational Data Governance. Krijg inzicht in het structureren van datateams om zowel centraal als lokaal aan uw behoeften te voldoen en leer hoe federated data governance kan zorgen voor verantwoording in de hele organisatie. We gaan in op een aantal uitdagingen op het gebied van data governance met betrekking tot dataproducten en het opstellen van datacontracten om de verwachtingen en verantwoordelijkheden van alle teams op elkaar af te stemmen.
Onderwerpen en discussiepunten:
- Data als bedrijfsmiddel – wat houdt dat in?
- Structureren van datateams voor flexibiliteit en impact
- Waarborgen van dataverantwoordelijkheid met duidelijk eigenaarschap
- Gefedereerde data governance implementeren met een balans tussen controle en autonomie
- Lange termijn handhaving van datamanagement toepassingen.
Data Modelling and Packaged Software
Concept Modelling for Business Analysts [English spoken]
Whether you call it a conceptual data model, a domain model, a business object model, or even a “thing model,” the concept model is seeing a worldwide resurgence of interest. Why? Because a concept model is a fundamental technique for improving communication among stakeholders in any sort of initiative. Sadly, that communication often gets lost – in the clouds, in the weeds, or in chasing the latest bright and shiny object. Having experienced this, Business Analysts everywhere are realizing Concept Modelling is a powerful addition to their BA toolkit. This session will even show how a concept model can be used to easily identify use cases, user stories, services, and other functional requirements.
Realizing the value of concept modelling is also, surprisingly, taking hold in the data community. “Surprisingly” because many data practitioners had seen concept modelling as an “old school” technique. Not anymore! In the past few years, data professionals who have seen their big data, data science/AI, data lake, data mesh, data fabric, data lakehouse, etc. efforts fail to deliver expected benefits realise it is because they are not based on a shared view of the enterprise and the things it cares about. That’s where concept modelling helps. Data management/governance teams are (or should be!) taking advantage of the current support for Concept Modelling. After all, we can’t manage what hasn’t been modelled!
The Agile community is especially seeing the need for concept modelling. Because Agile is now the default approach, even on enterprise-scale initiatives, Agile teams need more than some user stories on Post-its in their backlog. Concept modelling is being embraced as an essential foundation on which to envision and develop solutions. In all these cases, the key is to see a concept model as a description of a business, not a technical description of a database schema.
This workshop introduces concept modelling from a non-technical perspective, provides tips and guidelines for the analyst, and explores entity-relationship modelling at conceptual and logical levels using techniques that maximise client engagement and understanding. We’ll also look at techniques for facilitating concept modelling sessions (virtually and in-person), applying concept modelling within other disciplines (e.g., process change or business analysis,) and moving into more complex modelling situations.
Drawing on over forty years of successful consulting and modelling, on projects of every size and type, this session provides proven techniques backed up with current, real-life examples.
Topics include:
- The essence of concept modelling and essential guidelines for avoiding common pitfalls
- Methods for engaging our business clients in conceptual modelling without them realizing it
- Applying an easy, language-oriented approach to initiating development of a concept model
- Why bottom-up techniques often work best
- “Use your words!” – how definitions and assertions improve concept models
- How to quickly develop useful entity definitions while avoiding conflict
- Why a data model needs a sense of direction
- The four most common patterns in data modelling, and the four most common errors in specifying entities
- Making the transition from conceptual to logical using the world’s simplest guide to normalisation
- Understand “the four Ds of data modelling” – definition, dependency, demonstration, and detail
- Tips for conducting a concept model/data model review presentation
- Critical distinctions among conceptual, logical, and physical models
- Using concept models to discover use cases, business events, and other requirements
- Interesting techniques to discover and meet additional requirements
- How concept models help in package implementations, process change, and Agile development
Learning Objectives:
- Understand the essential components of a concept model – things (entities) facts about things (relationships and attributes) and rules
- Use entity-relationship modelling to depict facts and rules about business entities at different levels of detail and perspectives, specifically conceptual (overview) and logical (detailed) models
- Apply a variety of techniques that support the active participation and engagement of business professionals and subject matter experts
- Develop conceptual and logical models quickly using repeatable and Agile methods
- Draw an Entity-Relationship Diagram (ERD) for maximum readability
- Read a concept model/data model, and communicate with specialists using the appropriate terminology.
Data Mesh - Federated Data Governance: Structuring Teams and Driving Accountability
In today’s distributed and dynamic data landscapes, traditional approaches to governance and team organization can no longer keep pace. To unlock the full potential of data as a strategic asset, organizations must rethink how they manage, govern, and structure their data functions. This course, rooted in the principles of Federated Computational Data Governance, explores how to balance centralized oversight with distributed autonomy while ensuring accountability and alignment across teams.
Why We Need a New Approach
In many organizations, data governance is struggling to find its place, providing static policies focused on compliance rather than enablers of innovation. However, modern organizations need governance frameworks that are flexible, computational, and adaptive to distributed ecosystems. Federated data governance provides the balance needed to:
- Enable innovation through decentralized decision-making while maintaining control.
- Foster collaboration and alignment between central oversight and distributed teams
- Ensure accountability and ownership, even in complex, multi-team environments.
By introducing computational models and distributed governance principles, this course shows how to create a scalable, adaptable data team and framework.
The Three-Dimensional Approach to Structuring Data Teams
Data teams today must operate across three key dimensions to meet the demands of strategic alignment, operational execution, and distributed autonomy. Participants will learn how to organize their teams to:
- Strategic and Tactical Levels: Align data initiatives with organizational goals and ensure compliance with overarching governance frameworks.
- Operational Efficiency: Build robust processes, tools, and workflows to maintain data quality, security, and accessibility.
- Distributed Autonomy: Embed data functions into business units or regions, empowering them to act independently while adhering to shared principles.
This multi-layered approach ensures that data teams can balance innovation with foundational stability, creating a system that supports agility without sacrificing control.
Ensuring Data Accountability in Distributed Landscapes
As data becomes more distributed, accountability is critical to maintaining trust, quality, and compliance. The course will cover:
- Data Ownership and Stewardship: Defining clear roles and responsibilities for maintaining data quality and ethical use.
- Data Contracts: Establishing agreements between producers and consumers to clarify expectations, autonomy, and responsibilities.
- Creating a Culture of Responsibility: Ensuring that every team member understands their role in the data ecosystem, fostering a sense of ownership and trust.
Key Topics Covered
This course closely aligns with the workshop outline and includes practical, actionable insights into:
- Federated Data Governance: How to implement distributed authority while maintaining centralized oversight.
- Data Products and Data Contracts: Why design reusable, scalable data products and establish clear data contracts to streamline collaboration and accountability.
- Team Structures for Impact: Organizing data teams across strategic, operational, and distributed dimensions to maximize flexibility and innovation.
- Sustainability in Governance: Drawing lessons from long-term projects like NASA’s Mars Global Surveyor to ensure that governance systems are adaptable and maintainable over time.
Learning Objectives
- By the end of this course, participants will have a deep understanding of how to:
- Build and manage federated governance frameworks that balance autonomy and alignment
- Structure data teams to meet the dual needs of transformation and stability
- Embed accountability into every level of the organization through clear roles, data contracts, and a culture of ownership
- Implement sustainable practices that ensure long-term success in data management and governance.
Who is it for?
This course is designed for data leaders, managers, and governance professionals who want to create scalable and effective data organizations. Whether you’re responsible for strategy, compliance, or operations, you’ll gain tools and insights to navigate the evolving data landscape with confidence.
Detailed Workshop Outline
1. Introduction
Overview of Workshop Goals: Explain the importance of data as an asset and why organizations must move beyond treating data as just a service.
Solar System Metaphor: Introduce the concept of the data organization as a solar system, with data teams, governance, and accountability as key planetary bodies that need alignment for optimal performance.Key Points:
- Data as a core asset vs. a service
- The relationship between data, digital, and AI – why they aren’t interchangeable
- The balance between transformation and strong foundational structures in data management.Key Learning: Participants will understand why it’s essential to treat data as a core asset, setting the stage for exploring how to structure data teams and governance effectively.
2. Data Accountability: Creating a Culture of Ownership and Responsibility
Why Data Accountability Matters: Without clear accountability, data quality, security, and data availability suffer.
- The need for clarity in data ownership
- Creating a culture where team members feel responsible for data
- Defining clear data accountability and responsibility roles across the organization (Data Stewards, Data Owners, etc.).
Practical Steps to Ensure Accountability:
- Setting up reporting structures for data quality
- Understanding the value of Data Products and Data Contracts to codify accountability
- Implementing checks and balances for data privacy and security
- How to align individual accountability with organizational data goals.
Activity: Scenario-based discussion where participants identify where accountability is lacking in a fictional data-driven organization, and propose solutions for creating accountability.
Key Learning: Participants will gain insights into what data accountability entails, ensuring each team member knows their role in maintaining data quality and governance.
3. Data Governance Models: Federated Governance and Distributed Authority
Introduction to Data Governance: Why data governance is essential to manage risk, ensure compliance, and drive effective data use.
Federated Data Governance: What it is and how it works – balancing centralized oversight with distributed ownership across data hubs.
- The Gravitational Pull of strong governance: Central authority ensures alignment, while decentralized teams maintain autonomy.
- How to harmonize data governance policies across departments without losing agility.
Key Components of a Data Governance Framework:
- Roles and Responsibilities
- Data access controls and security measures
- Compliance with legal and ethical guidelines (e.g., GDPR)
- Continuous governance process for maintaining standards.
Activity: In groups, participants will design a federated governance model for a hypothetical organization, ensuring alignment between distributed teams and central governance.
Key Learning: Participants will learn how to implement a federated data governance model that balances control with autonomy, ensuring alignment across the organization.
4. Structuring Data Teams: Balancing Centralized and Distributed Needs
Discussion: Challenges in organizing data teams.
- Centralized vs. decentralized data functions
- Roles and responsibilities: What does a modern data team look like?
- Data Science, Data Engineering, DataOps, Data Management, etc.
- Balancing Innovation and Foundation: How do you organize a team that is both transformative (innovation-focused) and foundational (infrastructure-focused)?
Activity: Group exercise where participants design an ideal data team structure that addresses both distributed and centralized organizational needs.
Key Learning: Participants will learn how to create a data team structure that is flexible enough to meet both innovation-driven and operational demands.
5. Navigating Long-Term Sustainability: Lessons from NASA’s Mars Global Surveyor
Reflection: Insights from NASA’s Mars Global Surveyor and NASA’s Mars Climate Orbiter.
- Long-term data management challenges
- The importance of human involvement (Human-in-the-loop) in managing complex systems
- Sustainability in data practices: How to ensure that your data organization remains agile and maintainable over time.
Key Learning: Participants will leave with strategies for ensuring long-term sustainability and scalability in their data governance and team structures.
6. Wrap-Up and Key Takeaways
Summarizing the Journey: Recap of the solar system metaphor and how the workshop’s concepts apply to real-world data challenges.
Key Takeaways:
- How to structure data teams for maximum flexibility and impact
- Ensuring data accountability through clear roles and ownership
- Designing a federated data governance model to balance distributed autonomy with central oversight
- Practical steps to create a sustainable, future-proof data organization.
Q&A and Next Steps: Open the floor for final questions and discussions about how participants can implement the lessons in their own organizations.
Lees minder
Concept Modelling for Business Analysts [English spoken]
Whether you call it a conceptual data model, a domain model, a business object model, or even a “thing model,” the concept model is seeing a worldwide resurgence of interest. Why? Because a concept model is a fundamental technique for improving communication among stakeholders in any sort of initiative. Sadly, that communication often gets lost – in the clouds, in the weeds, or in chasing the latest bright and shiny object. Having experienced this, Business Analysts everywhere are realizing Concept Modelling is a powerful addition to their BA toolkit. This session will even show how a concept model can be used to easily identify use cases, user stories, services, and other functional requirements.
Realizing the value of concept modelling is also, surprisingly, taking hold in the data community. “Surprisingly” because many data practitioners had seen concept modelling as an “old school” technique. Not anymore! In the past few years, data professionals who have seen their big data, data science/AI, data lake, data mesh, data fabric, data lakehouse, etc. efforts fail to deliver expected benefits realise it is because they are not based on a shared view of the enterprise and the things it cares about. That’s where concept modelling helps. Data management/governance teams are (or should be!) taking advantage of the current support for Concept Modelling. After all, we can’t manage what hasn’t been modelled!
The Agile community is especially seeing the need for concept modelling. Because Agile is now the default approach, even on enterprise-scale initiatives, Agile teams need more than some user stories on Post-its in their backlog. Concept modelling is being embraced as an essential foundation on which to envision and develop solutions. In all these cases, the key is to see a concept model as a description of a business, not a technical description of a database schema.
This workshop introduces concept modelling from a non-technical perspective, provides tips and guidelines for the analyst, and explores entity-relationship modelling at conceptual and logical levels using techniques that maximise client engagement and understanding. We’ll also look at techniques for facilitating concept modelling sessions (virtually and in-person), applying concept modelling within other disciplines (e.g., process change or business analysis,) and moving into more complex modelling situations.
Drawing on over forty years of successful consulting and modelling, on projects of every size and type, this session provides proven techniques backed up with current, real-life examples.
Topics include:
- The essence of concept modelling and essential guidelines for avoiding common pitfalls
- Methods for engaging our business clients in conceptual modelling without them realizing it
- Applying an easy, language-oriented approach to initiating development of a concept model
- Why bottom-up techniques often work best
- “Use your words!” – how definitions and assertions improve concept models
- How to quickly develop useful entity definitions while avoiding conflict
- Why a data model needs a sense of direction
- The four most common patterns in data modelling, and the four most common errors in specifying entities
- Making the transition from conceptual to logical using the world’s simplest guide to normalisation
- Understand “the four Ds of data modelling” – definition, dependency, demonstration, and detail
- Tips for conducting a concept model/data model review presentation
- Critical distinctions among conceptual, logical, and physical models
- Using concept models to discover use cases, business events, and other requirements
- Interesting techniques to discover and meet additional requirements
- How concept models help in package implementations, process change, and Agile development
Learning Objectives:
- Understand the essential components of a concept model – things (entities) facts about things (relationships and attributes) and rules
- Use entity-relationship modelling to depict facts and rules about business entities at different levels of detail and perspectives, specifically conceptual (overview) and logical (detailed) models
- Apply a variety of techniques that support the active participation and engagement of business professionals and subject matter experts
- Develop conceptual and logical models quickly using repeatable and Agile methods
- Draw an Entity-Relationship Diagram (ERD) for maximum readability
- Read a concept model/data model, and communicate with specialists using the appropriate terminology.
Data Mesh - Federated Data Governance: Structuring Teams and Driving Accountability
In today’s distributed and dynamic data landscapes, traditional approaches to governance and team organization can no longer keep pace. To unlock the full potential of data as a strategic asset, organizations must rethink how they manage, govern, and structure their data functions. This course, rooted in the principles of Federated Computational Data Governance, explores how to balance centralized oversight with distributed autonomy while ensuring accountability and alignment across teams.
Why We Need a New Approach
In many organizations, data governance is struggling to find its place, providing static policies focused on compliance rather than enablers of innovation. However, modern organizations need governance frameworks that are flexible, computational, and adaptive to distributed ecosystems. Federated data governance provides the balance needed to:
- Enable innovation through decentralized decision-making while maintaining control.
- Foster collaboration and alignment between central oversight and distributed teams
- Ensure accountability and ownership, even in complex, multi-team environments.
By introducing computational models and distributed governance principles, this course shows how to create a scalable, adaptable data team and framework.
The Three-Dimensional Approach to Structuring Data Teams
Data teams today must operate across three key dimensions to meet the demands of strategic alignment, operational execution, and distributed autonomy. Participants will learn how to organize their teams to:
- Strategic and Tactical Levels: Align data initiatives with organizational goals and ensure compliance with overarching governance frameworks.
- Operational Efficiency: Build robust processes, tools, and workflows to maintain data quality, security, and accessibility.
- Distributed Autonomy: Embed data functions into business units or regions, empowering them to act independently while adhering to shared principles.
This multi-layered approach ensures that data teams can balance innovation with foundational stability, creating a system that supports agility without sacrificing control.
Ensuring Data Accountability in Distributed Landscapes
As data becomes more distributed, accountability is critical to maintaining trust, quality, and compliance. The course will cover:
- Data Ownership and Stewardship: Defining clear roles and responsibilities for maintaining data quality and ethical use.
- Data Contracts: Establishing agreements between producers and consumers to clarify expectations, autonomy, and responsibilities.
- Creating a Culture of Responsibility: Ensuring that every team member understands their role in the data ecosystem, fostering a sense of ownership and trust.
Key Topics Covered
This course closely aligns with the workshop outline and includes practical, actionable insights into:
- Federated Data Governance: How to implement distributed authority while maintaining centralized oversight.
- Data Products and Data Contracts: Why design reusable, scalable data products and establish clear data contracts to streamline collaboration and accountability.
- Team Structures for Impact: Organizing data teams across strategic, operational, and distributed dimensions to maximize flexibility and innovation.
- Sustainability in Governance: Drawing lessons from long-term projects like NASA’s Mars Global Surveyor to ensure that governance systems are adaptable and maintainable over time.
Learning Objectives
- By the end of this course, participants will have a deep understanding of how to:
- Build and manage federated governance frameworks that balance autonomy and alignment
- Structure data teams to meet the dual needs of transformation and stability
- Embed accountability into every level of the organization through clear roles, data contracts, and a culture of ownership
- Implement sustainable practices that ensure long-term success in data management and governance.
Who is it for?
This course is designed for data leaders, managers, and governance professionals who want to create scalable and effective data organizations. Whether you’re responsible for strategy, compliance, or operations, you’ll gain tools and insights to navigate the evolving data landscape with confidence.
Detailed Workshop Outline
1. Introduction
Overview of Workshop Goals: Explain the importance of data as an asset and why organizations must move beyond treating data as just a service.
Solar System Metaphor: Introduce the concept of the data organization as a solar system, with data teams, governance, and accountability as key planetary bodies that need alignment for optimal performance.Key Points:
- Data as a core asset vs. a service
- The relationship between data, digital, and AI – why they aren’t interchangeable
- The balance between transformation and strong foundational structures in data management.Key Learning: Participants will understand why it’s essential to treat data as a core asset, setting the stage for exploring how to structure data teams and governance effectively.
2. Data Accountability: Creating a Culture of Ownership and Responsibility
Why Data Accountability Matters: Without clear accountability, data quality, security, and data availability suffer.
- The need for clarity in data ownership
- Creating a culture where team members feel responsible for data
- Defining clear data accountability and responsibility roles across the organization (Data Stewards, Data Owners, etc.).
Practical Steps to Ensure Accountability:
- Setting up reporting structures for data quality
- Understanding the value of Data Products and Data Contracts to codify accountability
- Implementing checks and balances for data privacy and security
- How to align individual accountability with organizational data goals.
Activity: Scenario-based discussion where participants identify where accountability is lacking in a fictional data-driven organization, and propose solutions for creating accountability.
Key Learning: Participants will gain insights into what data accountability entails, ensuring each team member knows their role in maintaining data quality and governance.
3. Data Governance Models: Federated Governance and Distributed Authority
Introduction to Data Governance: Why data governance is essential to manage risk, ensure compliance, and drive effective data use.
Federated Data Governance: What it is and how it works – balancing centralized oversight with distributed ownership across data hubs.
- The Gravitational Pull of strong governance: Central authority ensures alignment, while decentralized teams maintain autonomy.
- How to harmonize data governance policies across departments without losing agility.
Key Components of a Data Governance Framework:
- Roles and Responsibilities
- Data access controls and security measures
- Compliance with legal and ethical guidelines (e.g., GDPR)
- Continuous governance process for maintaining standards.
Activity: In groups, participants will design a federated governance model for a hypothetical organization, ensuring alignment between distributed teams and central governance.
Key Learning: Participants will learn how to implement a federated data governance model that balances control with autonomy, ensuring alignment across the organization.
4. Structuring Data Teams: Balancing Centralized and Distributed Needs
Discussion: Challenges in organizing data teams.
- Centralized vs. decentralized data functions
- Roles and responsibilities: What does a modern data team look like?
- Data Science, Data Engineering, DataOps, Data Management, etc.
- Balancing Innovation and Foundation: How do you organize a team that is both transformative (innovation-focused) and foundational (infrastructure-focused)?
Activity: Group exercise where participants design an ideal data team structure that addresses both distributed and centralized organizational needs.
Key Learning: Participants will learn how to create a data team structure that is flexible enough to meet both innovation-driven and operational demands.
5. Navigating Long-Term Sustainability: Lessons from NASA’s Mars Global Surveyor
Reflection: Insights from NASA’s Mars Global Surveyor and NASA’s Mars Climate Orbiter.
- Long-term data management challenges
- The importance of human involvement (Human-in-the-loop) in managing complex systems
- Sustainability in data practices: How to ensure that your data organization remains agile and maintainable over time.
Key Learning: Participants will leave with strategies for ensuring long-term sustainability and scalability in their data governance and team structures.
6. Wrap-Up and Key Takeaways
Summarizing the Journey: Recap of the solar system metaphor and how the workshop’s concepts apply to real-world data challenges.
Key Takeaways:
- How to structure data teams for maximum flexibility and impact
- Ensuring data accountability through clear roles and ownership
- Designing a federated data governance model to balance distributed autonomy with central oversight
- Practical steps to create a sustainable, future-proof data organization.
Q&A and Next Steps: Open the floor for final questions and discussions about how participants can implement the lessons in their own organizations.
Lees minder
Liever online? Volg via de live stream!
Het congres kan zowel live in Utrecht als online worden gevolgd. Deelnemers aan het congres hebben bovendien nog enkele maanden toegang tot de video opnames dus als u een sessie moet missen, is er geen man overboord. Ook kunt u hierdoor alle parallelsessies achteraf nog bekijken.
2 april 2025
Zaal 1 Linda Terlouw
Zaal 1 Jos van Dongen
Zaal 1 Winfried Adalbert Etzel
workshops 2025
3 april, Workshops Winfried Adalbert Etzel