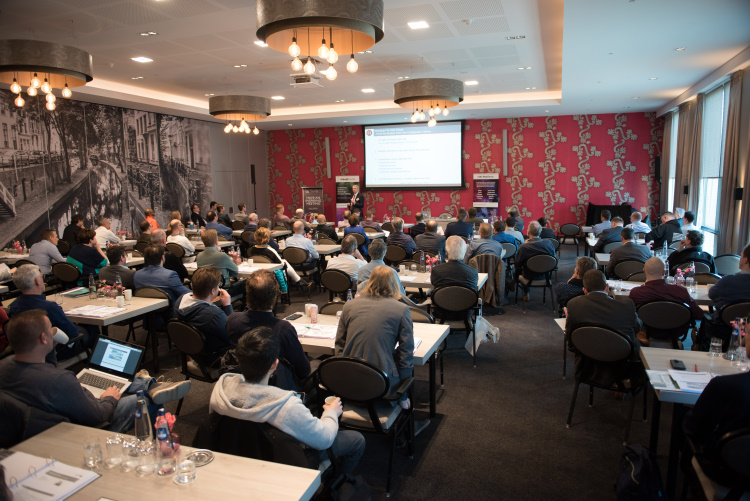
[DE WORKSHOPS OP 28 MAART ZIJN ENKEL LIVE IN UTRECHT.]
Uw dagvoorzitter
Data Architecture Evolution and the Impact on Analytics [Engelstalig]
In the last 12-18 months we have seen many different architectures emerge from many different vendors who claim to be offering ‘the modern data architecture solution’ for the data-driven enterprise. These range from streaming data platforms to data lakes, to cloud data warehouses supporting structured, semi-structured and unstructured data, cloud data warehouses supporting external tables and federated query processing, lakehouses, data fabric, and federated query platforms offering virtual views of data and virtual data products on data in data lakes and lakehouses. In addition, all of these vendor architectures are claiming to support the building of data products in a data mesh. It’s not surprising therefore, that customers are confused as to which option to choose.
However, in 2023, key changes have emerged including much broader support for open table formats such as Apache Iceberg, Apache Hudi and Delta Lake in many other vendor data platforms. In addition, we have seen significant new milestones in extending the ISO SQL Standard to support new kinds of analytics in general purpose SQL. Also, AI has also advanced to work across any type of data.
The key question is what does this all mean for data management? What is the impact of this on analytical data platforms and what does it mean for customers? This session looks at this evolution and helps customers realise the potential of what’s now possible and how they can exploit it for competitive advantage.
- The demand for data and AI
- The need for a data foundation to underpin data and AI initiatives
- The emergence of data mesh and data products
- The challenge of a distributed data estate
- Data fabric and how can they help build data products
- Data architecture options for building data products
- The impact of open table formats and query language extensions on architecture modernisation
- Is the convergence of analytical workloads possible?
Connecting Meaning: The promise and challenges of Knowledge Graphs as providers of large-scale data semantics [Engelstalig]
Ever since Google announced that “their knowledge graph allowed searching for things, not strings”, the term “knowledge graph” has been widely adopted, to denote any graph-like network of interrelated typed entities and concepts that can be used to integrate, share and exploit data and knowledge.
This idea of interconnected data under common semantics is actually much older and the term is a rebranding of several other concepts and research areas (semantic networks, knowledge bases, ontologies, semantic web, linked data etc). Google popularized this idea and made it more visible to the public and the industry, the result being several prominent companies, developing and using their own knowledge graphs for data integration, data analytics, semantic search, question answering and other cognitive applications.
As the use of knowledge graphs continues to expand across various domains, the need for ensuring the accuracy, reliability, and consensus of semantic information becomes paramount. The intricacies involved in constructing and utilizing knowledge graphs present a spectrum of challenges, from data quality assurance to ensuring scalability and adaptability to evolving contexts.
In this talk, we will delve deeper into the significance of knowledge graphs as facilitators of large-scale data semantics. The discussion will encompass the core concepts, challenges, and strategic considerations that architects and decision-makers encounter while initiating and implementing knowledge graph projects.
The session will cover:
- Understanding Knowledge Graphs: Exploring the fundamental concepts and significance of knowledge graphs in integrating, organizing, and harnessing data across diverse domains
- Challenges in Building Knowledge Graphs: Identifying and dissecting primary hurdles such as data quality assurance, schema alignment, scalability, and ongoing maintenance
- Strategic Dilemmas: Examining critical decision points and dilemmas faced by architects and executives when designing and executing knowledge graph initiatives
- Crafting an Effective Strategy: Outlining guidelines to formulate a robust knowledge graph strategy tailored to specific organizational goals, considering scalability, interoperability, and domain relevance.
Hybrid Query Processing in MotherDuck [Engelstalig]
MotherDuck is een nieuwe dienst die DuckDB met de cloud verbindt. Het product introduceert het concept van “hybrid query processing”: de mogelijkheid om query’s deels op de client en deels in de cloud uit te voeren. Deze lezing behandelt de motivatie voor MotherDuck en enkele van de gebruikssituaties, evenals de belangrijkste kenmerken van de systeemarchitectuur, die in hoge mate gebruik maakt van de uitbreidingsmechanismen van DuckDB. Om context te bieden, zal deze sessie ook een kort overzicht geven van de DuckDB architectuur.
- DuckDB
- Geschiedenis: MonetDB, VectorWise, Snowflake
- MotherDuck: DuckDB in de cloud
- Hybrid Query Processing
- Applications: Datateams en low-latency web analytics
Generative AI in Data Management and Analytics – A New Era of Assistance, Productivity and Automation [Engelstalig]
The emergence of generative AI has been described as a major breakthrough in technology. It has reduced the time to create new content and triggered a new wave of innovation that is impacting almost every type of software. New tools, applications and functionality are already emerging that are dramatically improving productivity, simplifying user experiences and paving the way for new ways of working. In this keynote session, Mike Ferguson, Europe’s leading IT industry analyst on Data Management and Analytics, looks at the impact generative AI is having on Data Management, BI and Data Science and what it can do to help shorten time to value.
- What is generative AI?
- What are the business benefits of generative AI?
- How is generative AI being used in data management?
- How is generative AI being used in data science and BI
- What does this mean for business going forward?
- What should you do to get started?
Democratisering van Data: Het Kwadrantenmodel in Actie
Traditioneel zijn datawarehouses primair ontworpen voor het oplossen van analysevraagstukken. Met de opkomst van data-democratisering groeit de behoefte om data breder binnen organisaties in te zetten. Dataconsumenten willen de beschikbare gegevens vrijer benutten, en historische data in datawarehouses wordt steeds waardevoller als bron voor het trainen van AI-modellen. In dit evoluerende landschap wordt het integreren van privacy by design in de architectuur essentieel. Het moet niet langer worden gezien als een hindernis, maar eerder als een katalysator voor deze vooruitgang. Het kwadrantenmodel van Damhof biedt hierbij een leidraad. Door deze benadering toe te passen, ontstaat niet alleen de mogelijkheid om te voldoen aan de groeiende eisen van dataconsumptie en AI-ontwikkelingen, maar leggen we ook een solide basis waarop innovatie wordt gestimuleerd.
– Datawarehouses en de rol binnen datascience
– Privacy by Design als katalysator
– Kwadrantenmodel in combinatie met datavirtualisatie
– Kostenreductie van experimenten.
Lunchpauze
Mixed Source Data Engineering en Analytics: het beste van twee werelden
De Erasmus Universiteit Rotterdam is een van de grootste academische instellingen van het land met als missie ‘het creëren van een positieve maatschappelijke impact’ en waar de Sustainable Development Goals van de Verenigde Naties als kompas dienen voor zowel onderzoek als onderwijs. Met de verscheidenheid en diversiteit aan onderwerpen binnen EUR is een open, flexibele, betaalbare en eenvoudig te gebruiken data & analytics-oplossing essentieel om data & AI-projecten te ondersteunen. Tegelijkertijd zijn er veel interne en externe factoren waarmee rekening moet worden gehouden: de overstap naar en migratie naar cloudoplossingen, de drang naar open science en open source, een steeds sneller veranderend technologielandschap en tot slot de adembenemende snelheid waarmee AI-oplossingen op de markt komen. Het maken van toekomstbestendige keuzes in deze omgeving is een ontmoedigende taak. Toch zijn er keuzes gemaakt en deze bestaan uit een mix van open source en eigen oplossingen, zowel on-premise als in de cloud, en worden geleid door moderne software engineering principes. Deze sessie zal het volgende belichten:
- De invloed van moderne software-engineeringprincipes zoals CI/CD op data-engineering, data management en analytics
- Hoe onafhankelijk te blijven en te voorkomen dat je vastzit aan een leverancier of cloudprovider
- De afweging tussen het bouwen, kopen en huren van hard- en software
- Hoe te standaardiseren op tools en technologie en tegelijkertijd flexibel te blijven.
Data Governance as Keystone for Compliant AI and Digital Trust [Engelstalig]
Data governance is the process of managing the availability, usability, integrity, and security of data in an organization. It is essential for ensuring that data is used ethically, responsibly, and in compliance with regulations and standards. Data governance also enables the development and deployment of AI systems that are aligned with the values, goals, and expectations of the stakeholders and the society. In this keynote, we will discuss how data governance can serve as a keystone for building ethical AI and digital trust. We will explore the challenges and opportunities of data governance in the context of AI, and present some best practices and frameworks for implementing data governance in AI projects. We will also share some examples and case studies of how data governance can help achieve ethical AI and digital trust outcomes. The keynote will conclude with some recommendations and future directions for data governance in the AI era.
By the end of this session, you will be able to:
- Define data governance and its importance for data and AI systems
- Identify the challenges and opportunities of data governance in the context of AI
- How to apply best practices and frameworks for data governance, such as data lifecycle management, data stewardship, data ethics principles, and data audit and assessment
- Explain how data governance can support ethical AI and digital trust outcomes, such as fairness, privacy, explainability, and reliability
- Recognize the roles and responsibilities of various actors and stakeholders in the AI ecosystem for data governance.
Data Mesh Light – getting there, step by step, avoiding the Mess [Engelstalig]
The Data Mesh approach has been well on its way as an alternative data management approach that does justice to the federative nature of most organizations and the need to provide ownership of data as close as possible to the business domains – where data is actually created and used. However, the transformational impact of Data Mesh is potentially big, and many organizations have found it difficult to implement the approach in all of its dimensions at once. Why not take a lighter approach, reaping benefits one by one, rather than going for an unprepared, deep dive into the Data Mesh rabbit hole?
- Recap: the key elements of the Data Mesh approach
- Best and worst practices from real life
- Crafting a step-by-step approach
- Architectural and technological considerations
- Adding semantics to the Data Mesh
- Using generative AI to augment a Data Mesh.
Concept Modelling and The Data-Process Connection [Engelstalig]
Whether you call it a conceptual data model, a domain map, a business object model, or even a “thing model,” a concept model is invaluable to process and architecture initiatives. Why? Because processes, capabilities, and solutions act on “things” – Settle Claim, Register Unit, Resolve Service Issue, and so on. Those things are usually “entities” or “objects” in the concept model, and clarity on “what is one of these things?” contributes immensely to clarity on what the corresponding processes are.
After introducing methods to get people, even C-level executives, engaged in concept modelling, we’ll introduce and get practice with guidelines to ensure proper naming and definition of entities/concepts/business objects. We’ll also see that success depends on recognising that a concept model is a description of a business, not a description of a database. Another key – don’t call it a data model!
Drawing on almost forty years of successful modelling, on projects of every size and type, this session introduces proven techniques backed up with current, real-life examples. Topics include:
- Concept modelling essentials – things, facts about things, and the policies and rules governing things
- “Guerrilla modelling” – how to get started on concept modelling without anyone realising it
- Naming conventions and graphic guidelines – ensuring correctness, consistency, and readability
- Concept models as a starting point for process discovery
- Practical examples of concept modelling supporting process work, architecture work, and commercial software selection.
Borrel
Uw dagvoorzitter
Data Architecture Evolution and the Impact on Analytics [Engelstalig]
In the last 12-18 months we have seen many different architectures emerge from many different vendors who claim to be offering ‘the modern data architecture solution’ for the data-driven enterprise. These range from streaming data platforms to data lakes, to cloud data warehouses supporting structured, semi-structured and unstructured data, cloud data warehouses supporting external tables and federated query processing, lakehouses, data fabric, and federated query platforms offering virtual views of data and virtual data products on data in data lakes and lakehouses. In addition, all of these vendor architectures are claiming to support the building of data products in a data mesh. It’s not surprising therefore, that customers are confused as to which option to choose.
However, in 2023, key changes have emerged including much broader support for open table formats such as Apache Iceberg, Apache Hudi and Delta Lake in many other vendor data platforms. In addition, we have seen significant new milestones in extending the ISO SQL Standard to support new kinds of analytics in general purpose SQL. Also, AI has also advanced to work across any type of data.
The key question is what does this all mean for data management? What is the impact of this on analytical data platforms and what does it mean for customers? This session looks at this evolution and helps customers realise the potential of what’s now possible and how they can exploit it for competitive advantage.
- The demand for data and AI
- The need for a data foundation to underpin data and AI initiatives
- The emergence of data mesh and data products
- The challenge of a distributed data estate
- Data fabric and how can they help build data products
- Data architecture options for building data products
- The impact of open table formats and query language extensions on architecture modernisation
- Is the convergence of analytical workloads possible?
Connecting Meaning: The promise and challenges of Knowledge Graphs as providers of large-scale data semantics [Engelstalig]
Ever since Google announced that “their knowledge graph allowed searching for things, not strings”, the term “knowledge graph” has been widely adopted, to denote any graph-like network of interrelated typed entities and concepts that can be used to integrate, share and exploit data and knowledge.
This idea of interconnected data under common semantics is actually much older and the term is a rebranding of several other concepts and research areas (semantic networks, knowledge bases, ontologies, semantic web, linked data etc). Google popularized this idea and made it more visible to the public and the industry, the result being several prominent companies, developing and using their own knowledge graphs for data integration, data analytics, semantic search, question answering and other cognitive applications.
As the use of knowledge graphs continues to expand across various domains, the need for ensuring the accuracy, reliability, and consensus of semantic information becomes paramount. The intricacies involved in constructing and utilizing knowledge graphs present a spectrum of challenges, from data quality assurance to ensuring scalability and adaptability to evolving contexts.
In this talk, we will delve deeper into the significance of knowledge graphs as facilitators of large-scale data semantics. The discussion will encompass the core concepts, challenges, and strategic considerations that architects and decision-makers encounter while initiating and implementing knowledge graph projects.
The session will cover:
- Understanding Knowledge Graphs: Exploring the fundamental concepts and significance of knowledge graphs in integrating, organizing, and harnessing data across diverse domains
- Challenges in Building Knowledge Graphs: Identifying and dissecting primary hurdles such as data quality assurance, schema alignment, scalability, and ongoing maintenance
- Strategic Dilemmas: Examining critical decision points and dilemmas faced by architects and executives when designing and executing knowledge graph initiatives
- Crafting an Effective Strategy: Outlining guidelines to formulate a robust knowledge graph strategy tailored to specific organizational goals, considering scalability, interoperability, and domain relevance.
Hybrid Query Processing in MotherDuck [Engelstalig]
MotherDuck is een nieuwe dienst die DuckDB met de cloud verbindt. Het product introduceert het concept van “hybrid query processing”: de mogelijkheid om query’s deels op de client en deels in de cloud uit te voeren. Deze lezing behandelt de motivatie voor MotherDuck en enkele van de gebruikssituaties, evenals de belangrijkste kenmerken van de systeemarchitectuur, die in hoge mate gebruik maakt van de uitbreidingsmechanismen van DuckDB. Om context te bieden, zal deze sessie ook een kort overzicht geven van de DuckDB architectuur.
- DuckDB
- Geschiedenis: MonetDB, VectorWise, Snowflake
- MotherDuck: DuckDB in de cloud
- Hybrid Query Processing
- Applications: Datateams en low-latency web analytics
Generative AI in Data Management and Analytics – A New Era of Assistance, Productivity and Automation [Engelstalig]
The emergence of generative AI has been described as a major breakthrough in technology. It has reduced the time to create new content and triggered a new wave of innovation that is impacting almost every type of software. New tools, applications and functionality are already emerging that are dramatically improving productivity, simplifying user experiences and paving the way for new ways of working. In this keynote session, Mike Ferguson, Europe’s leading IT industry analyst on Data Management and Analytics, looks at the impact generative AI is having on Data Management, BI and Data Science and what it can do to help shorten time to value.
- What is generative AI?
- What are the business benefits of generative AI?
- How is generative AI being used in data management?
- How is generative AI being used in data science and BI
- What does this mean for business going forward?
- What should you do to get started?
Democratisering van Data: Het Kwadrantenmodel in Actie
Traditioneel zijn datawarehouses primair ontworpen voor het oplossen van analysevraagstukken. Met de opkomst van data-democratisering groeit de behoefte om data breder binnen organisaties in te zetten. Dataconsumenten willen de beschikbare gegevens vrijer benutten, en historische data in datawarehouses wordt steeds waardevoller als bron voor het trainen van AI-modellen. In dit evoluerende landschap wordt het integreren van privacy by design in de architectuur essentieel. Het moet niet langer worden gezien als een hindernis, maar eerder als een katalysator voor deze vooruitgang. Het kwadrantenmodel van Damhof biedt hierbij een leidraad. Door deze benadering toe te passen, ontstaat niet alleen de mogelijkheid om te voldoen aan de groeiende eisen van dataconsumptie en AI-ontwikkelingen, maar leggen we ook een solide basis waarop innovatie wordt gestimuleerd.
– Datawarehouses en de rol binnen datascience
– Privacy by Design als katalysator
– Kwadrantenmodel in combinatie met datavirtualisatie
– Kostenreductie van experimenten.
Lunchpauze
Mixed Source Data Engineering en Analytics: het beste van twee werelden
De Erasmus Universiteit Rotterdam is een van de grootste academische instellingen van het land met als missie ‘het creëren van een positieve maatschappelijke impact’ en waar de Sustainable Development Goals van de Verenigde Naties als kompas dienen voor zowel onderzoek als onderwijs. Met de verscheidenheid en diversiteit aan onderwerpen binnen EUR is een open, flexibele, betaalbare en eenvoudig te gebruiken data & analytics-oplossing essentieel om data & AI-projecten te ondersteunen. Tegelijkertijd zijn er veel interne en externe factoren waarmee rekening moet worden gehouden: de overstap naar en migratie naar cloudoplossingen, de drang naar open science en open source, een steeds sneller veranderend technologielandschap en tot slot de adembenemende snelheid waarmee AI-oplossingen op de markt komen. Het maken van toekomstbestendige keuzes in deze omgeving is een ontmoedigende taak. Toch zijn er keuzes gemaakt en deze bestaan uit een mix van open source en eigen oplossingen, zowel on-premise als in de cloud, en worden geleid door moderne software engineering principes. Deze sessie zal het volgende belichten:
- De invloed van moderne software-engineeringprincipes zoals CI/CD op data-engineering, data management en analytics
- Hoe onafhankelijk te blijven en te voorkomen dat je vastzit aan een leverancier of cloudprovider
- De afweging tussen het bouwen, kopen en huren van hard- en software
- Hoe te standaardiseren op tools en technologie en tegelijkertijd flexibel te blijven.
Data Governance as Keystone for Compliant AI and Digital Trust [Engelstalig]
Data governance is the process of managing the availability, usability, integrity, and security of data in an organization. It is essential for ensuring that data is used ethically, responsibly, and in compliance with regulations and standards. Data governance also enables the development and deployment of AI systems that are aligned with the values, goals, and expectations of the stakeholders and the society. In this keynote, we will discuss how data governance can serve as a keystone for building ethical AI and digital trust. We will explore the challenges and opportunities of data governance in the context of AI, and present some best practices and frameworks for implementing data governance in AI projects. We will also share some examples and case studies of how data governance can help achieve ethical AI and digital trust outcomes. The keynote will conclude with some recommendations and future directions for data governance in the AI era.
By the end of this session, you will be able to:
- Define data governance and its importance for data and AI systems
- Identify the challenges and opportunities of data governance in the context of AI
- How to apply best practices and frameworks for data governance, such as data lifecycle management, data stewardship, data ethics principles, and data audit and assessment
- Explain how data governance can support ethical AI and digital trust outcomes, such as fairness, privacy, explainability, and reliability
- Recognize the roles and responsibilities of various actors and stakeholders in the AI ecosystem for data governance.
Data Mesh Light – getting there, step by step, avoiding the Mess [Engelstalig]
The Data Mesh approach has been well on its way as an alternative data management approach that does justice to the federative nature of most organizations and the need to provide ownership of data as close as possible to the business domains – where data is actually created and used. However, the transformational impact of Data Mesh is potentially big, and many organizations have found it difficult to implement the approach in all of its dimensions at once. Why not take a lighter approach, reaping benefits one by one, rather than going for an unprepared, deep dive into the Data Mesh rabbit hole?
- Recap: the key elements of the Data Mesh approach
- Best and worst practices from real life
- Crafting a step-by-step approach
- Architectural and technological considerations
- Adding semantics to the Data Mesh
- Using generative AI to augment a Data Mesh.
Concept Modelling and The Data-Process Connection [Engelstalig]
Whether you call it a conceptual data model, a domain map, a business object model, or even a “thing model,” a concept model is invaluable to process and architecture initiatives. Why? Because processes, capabilities, and solutions act on “things” – Settle Claim, Register Unit, Resolve Service Issue, and so on. Those things are usually “entities” or “objects” in the concept model, and clarity on “what is one of these things?” contributes immensely to clarity on what the corresponding processes are.
After introducing methods to get people, even C-level executives, engaged in concept modelling, we’ll introduce and get practice with guidelines to ensure proper naming and definition of entities/concepts/business objects. We’ll also see that success depends on recognising that a concept model is a description of a business, not a description of a database. Another key – don’t call it a data model!
Drawing on almost forty years of successful modelling, on projects of every size and type, this session introduces proven techniques backed up with current, real-life examples. Topics include:
- Concept modelling essentials – things, facts about things, and the policies and rules governing things
- “Guerrilla modelling” – how to get started on concept modelling without anyone realising it
- Naming conventions and graphic guidelines – ensuring correctness, consistency, and readability
- Concept models as a starting point for process discovery
- Practical examples of concept modelling supporting process work, architecture work, and commercial software selection.
Borrel
Data Products – From Design, to Build, to Publishing and Consumption [English spoken]
Most companies today are storing data and running applications in a hybrid multi-cloud environment. Analytical systems tend to be centralised and siloed like data warehouses and data marts for BI, cloud storage data lakes for data science and stand-alone streaming analytical systems for real-time analysis. These centralised systems rely on data engineers and data scientists working within each silo to ingest data from many different sources and engineer it for use in a specific analytical system or machine learning models. There are many issues with this centralised, siloed approach including multiple tools to prepare and integrate data, reinvention of data integration pipelines in each silo and centralised data engineering with poor understanding of source data unable to keep pace with business demands for new data.
To address these issues, a new approach called Data Mesh emerged in late 2019 attempting to accelerate creation of data for use in multiple analytical workloads. Data Mesh is a decentralised business domain-oriented approach to data ownership and data engineering to create a mesh of reusable data products that can be created once and shared across multiple analytical systems and workloads.
This half-day workshop looks at the development of data products in detail and also, how can you use a data marketplace to share and govern the sharing of data products across the enterprise to shorten time to value.
Learning Objectives:
- Strengths and weaknesses of centralised data architectures used in analytics
- The problems caused in existing analytical systems by a hybrid, multi-cloud data landscape
- The emergence of data mesh and data products
- What exactly a data product is and the types of data products that you can create
- The benefits that data products offer and what are the implementation options?
- How to organise to create data products in a decentralised environment so you avoid chaos?
- How business glossaries can help ensure data products are formally defined, understood by business users and semantically linked
- The critical importance of a data catalog in understanding what data is available
- What software is required to build, operate and govern a data mesh of data products for use in a data lake, a data lakehouse or data warehouse?
- What is data fabric software, how does it integrate with data catalogs and connect to data in your data estate
- An Implementation methodology to produce ready-made, trusted, reusable data products
- Collaborative domain-oriented development of modular and distributed DataOps pipelines to create data products
- How a data catalog and automation software can be used to generate DataOps pipelines
- Managing data quality, privacy, access security, versioning, and the lifecycle of data products
- Publishing semantically linked data products in a data marketplace for others to consume and use
- Governing the sharing and use of data products in a data marketplace
- Consuming data products in an MDM system
- Consuming and assembling data products in multiple analytical systems like data warehouses, lakehouses and graph databases to shorten time to value.
Who is it for?
This seminar is intended for business data analysts, data architects, chief data officers, master data management professionals, data scientists, IT ETL developers, and data governance professionals. It assumes you understand basic data management principles and data architecture plus a reasonable understanding of data cleansing, data integration, data catalogs, data lakes and data governance.
Detailed course outline
Most companies today are storing data and running applications in a hybrid multi-cloud environment. Analytical systems tend to be centralised and siloed like data warehouses and data marts for BI, cloud storage data lakes or Hadoop for data science and stand-alone streaming analytical systems for real-time analysis. These centralised systems rely on data engineers and data scientists working within each silo to ingest data from many different sources, clean and integrate it for use in a specific analytical system or machine learning models. There are many issues with this centralised, siloed approach including multiple tools to prepare and integrate data, reinvention of data integration pipelines in each silo and centralised data engineering with poor understanding of source data unable to keep pace with business demands for new data. Also, master data is not well managed.
To address these issues, a new approach emerged in late 2019 attempting to accelerate creation of data for use in multiple analytical workloads. That approach is Data Mesh. Data Mesh is a decentralised business domain-oriented approach to data ownership and data engineering to create a mesh of reusable data products that can be created once and shared across multiple analytical systems and workloads. A Data Mesh can be implemented in a number of ways. These include using one or more cloud storage accounts on cloud storage, on an organised data lake, on a Lakehouse, on a data cloud, using Kafka or using data virtualisation. Data products can then be consumed in other pipelines for use in streaming analytics, Data Warehouses or Lakehouse Gold Tables, for use in business intelligence, feature stores for use data science, graph databases for use in graph analysis and other analytical workloads.
This half-day workshop looks at the development of data products in detail. It also looks at the strengths and weaknesses of data mesh implementation options for data product development. Which architecture is best to implement this? How do you co-ordinate multiple domain-oriented teams and use common data infrastructure software like Data Fabric to create high-quality, compliant, reusable, data products in a Data Mesh. Is there a methodology for creating data products? Also, how can you use a data marketplace to share and govern the sharing of data products? The objective is to shorten time to value while also ensuring that data is correctly governed and engineered in a decentralised environment. It also looks at the organisational implications of Data Mesh and how to create sharable data products for use as master data, in a data warehouse, in data science, in graph analysis and in real-time streaming analytics to drive business value? Technologies discussed includes data catalogs, data fabric for collaborative development of data integration pipelines to create data products, DataOps to speed up the process, data orchestration automation, data observability and data marketplaces.
- What are data products?
- What makes creating data products different from other approaches to creating data for use analytical workloads?
- A best practice methodology for creating data products
- How to design semantically linked data products to enable rapid consumption and use of data to produce new insights
- Quick start mechanisms to speed up data product design
- Defining common business data names for data products in a business glossary
- Data modelling techniques for data products
- Discovering data needed to build data products using a data catalog
- Developing DataOps pipelines to engineer the data needed using data fabric
- Publishing data products – the role of the data marketplace
- Governing access to and use of data products across the enterprise
- Consuming and assembling data products for use in multiple analytical workloads
- Technologies and skills needed.
Knowledge Graphs - pragmatische aanpak en best practices [English spoken]
In today’s data-driven landscape, the concept of a knowledge graph has emerged as a pivotal framework for managing and utilizing interconnected data and information. Stemming from Google’s proclamation that shifted the focus from searching for strings to understanding entities and relationships, the term encapsulates a network of interconnected entities and concepts, facilitating data integration, sharing, and utilization within organizations.
Amid the widespread adoption of knowledge graphs across diverse domains, ensuring the accuracy, reliability, and consensus of semantic information becomes an imperative. The construction and utilization of these graphs present multifaceted challenges, ranging from ensuring data quality to scaling and adapting to evolving contexts.
Implementing a successful Knowledge Graph initiative within an organization demands strategic decisions before and during its execution. Often overlooked are critical considerations such as managing trade-offs between knowledge quality and other factors, prioritizing knowledge evolution, and allocating resources effectively. Neglecting these facets can lead to friction and suboptimal outcomes.
This half-day seminar delves into the technical, business, and organizational dimensions essential for data practitioners and executives embarking on a Knowledge Graph initiative. Offering insights gleaned from real-world case studies, the seminar provides a comprehensive framework that combines cutting-edge techniques with pragmatic advice. It equips participants to navigate the complexities of executing a knowledge graph project successfully.
Moreover, the session addresses pivotal strategic dilemmas encountered during the design and execution phases of knowledge graph projects, and outlines potential approaches to tackle these challenges, empowering attendees with actionable strategies to optimize their initiatives.
Learning Objectives
- Understand the key factors determining the feasibility and viability of implementing a knowledge graph in an organization.
- Identify and articulate the fundamental questions crucial for preparing and launching a successful knowledge graph initiative.
- Learn techniques to determine and prioritize the content requirements of a knowledge graph.
- Grasp best practices in schema design for knowledge graphs, addressing real-world challenges of uncertainty and vagueness.
- Explore strategies and guidelines for populating a knowledge graph, evaluating available knowledge extraction systems.
- Gain insights into assessing and prioritizing quality dimensions within a knowledge graph.
- Explore practical applications of knowledge graphs, such as entity disambiguation and semantic search, optimizing performance through design principles.
- Gain insights into methodologies for ongoing maintenance and evolution of knowledge graphs, ensuring their sustained relevance and adaptability across time.
Who is it for?
- Data practitioners: Data scientists, data engineers, data analysts, and database administrators seeking to deepen their understanding of knowledge graphs, their implementation, and the technical intricacies involved.
- Technology Leaders: Architects, CTOs , and IT professionals exploring or leading initiatives involving data integration, semantic technologies, and knowledge management systems.
- Business Executives and Managers: Leaders and decision-makers responsible for overseeing data strategies, innovation, and organizational transformation, aiming to comprehend the strategic implications and business value derived from knowledge graph initiatives.
Course Outline
The seminar will walk participants through 8 key stages of introducing, developing, delivering and evolving Knowledge Graphs in an organization. These are:
Stage 1 – “Knowing where you are getting into”
- Clarification of the knowledge graph concept
- Key factors influencing the ease or difficulty of building a knowledge graph
- Evaluating feasibility and viability of implementing a knowledge graph in a specific organization and for a particular business problem
Stage 2 – ”Setting up the stage”
- Exploring 5 key questions essential before initiating knowledge graph development
- Defining what, why, how, who, and the stakeholders involved in the project
- Outlining actions required to seek and discover answers to these questions
Stage 3 – “Deciding what to build”:
- Delving into knowledge graph specification
- Use of competency questions for gap analysis between organizational knowledge capabilities and needs
- Scoping and prioritizing knowledge graph content
Stage 4 – “Giving it a shape”
- Schema design using Ontology Representation and Engineering
- Identification of conceptual modeling best practices, dilemmas, and pitfalls
- Addressing uncertainty and vagueness
Stage 5 – “Giving it substance”
- Exploring the challenging task of knowledge graph population
- Description of population tasks and associated difficulties
- Designing optimal population pipelines
Stage 6 – “Ensuring it’s good”:
- Assessing knowledge graph quality, defining dimensions, and metrics
- Insights into quality trade-offs and prioritization of dimensions
- Measuring quality and effective prioritization of focus areas
Stage 7 – “Making it useful”:
- Typical knowledge graph applications
- Guidelines and best practices for optimizing knowledge graph usefulness and value
Stage 8 – “Making it last”:
- Addressing the challenge of knowledge graph maintenance and evolution
- Detecting, measuring, and monitoring concept drift
- Best practices for enabling continuous improvement and preventing knowledge graph obsolescence over time.
Liever online? Volg via de live stream!
Het congres kan zowel live in Utrecht als online worden gevolgd. Deelnemers aan het congres hebben bovendien nog enkele maanden toegang tot de video opnames dus als u een sessie moet missen, is er geen man overboord. Ook kunt u hierdoor alle parallelsessies achteraf nog bekijken.
27 maart 2024
Zaal 1 Mike Ferguson
Zaal 1 Panos Alexopoulos
Zaal 1 Mike Ferguson
Plenair
Zaal 1 Jos van Dongen
Zaal 2 Jan Henderyckx
Zaal 1 Ron Tolido
Workshops
28 maart Mike Ferguson
28 maart Panos Alexopoulos